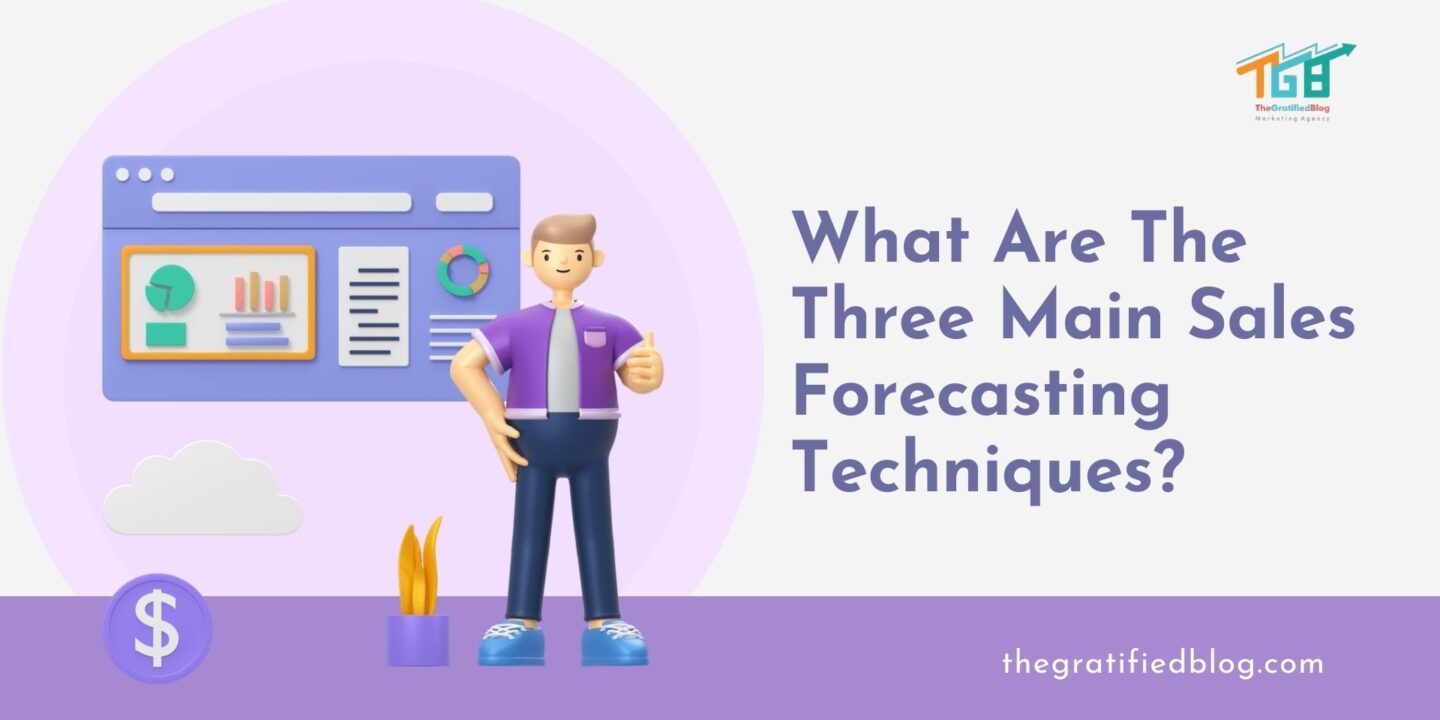
Sales forecasting is crucial to any business, as it helps companies predict future sales and plan accordingly. There are three main sales forecasting techniques that organizations can utilize to predict future sales with more accuracy. Each method has its own strengths and weaknesses, and understanding how to implement them effectively can help businesses make better-informed decisions. This article, “What are the three main sales forecasting techniques?” will delve deeper into these three techniques and explore their relevance in today’s competitive business landscape.
Understanding Sales Forecasting

Sales forecasting is the process of predicting future sales performance based on historical data, market trends, and various influencing factors. It involves analyzing past sales patterns, economic indicators, customer behavior, and competitor activity to estimate future sales volumes, revenue, and potential challenges.
Sales forecasting helps businesses anticipate demand, allocate resources effectively, set achievable targets, and make informed decisions regarding production, inventory, and marketing strategy. Providing insights into potential sales outcomes enables businesses to adapt and plan accordingly, optimizing their operations and maximizing profitability.
Importance Of Sales Forecasting In Business
Sales forecasting plays a vital role in businesses’ strategic decision-making. Businesses can better allocate resources, plan inventory levels, and adjust marketing strategies by accurately predicting future sales. This foresight enables organizations to optimize operations, reduce costs, and maximize profits.
Moreover, sales forecasting helps set realistic goals, evaluate performance, and identify improvement areas. With the proper forecasting techniques, businesses can stay ahead of market trends and adjust to evolving consumer habits while preserving a competitive advantage within the sector.
What Are The Three Main Sales Forecasting Techniques?
Forecasting sales accurately is crucial for strategic planning, resource allocation, and overall success in the dynamic business realm. Whether a startup or an established enterprise, a solid grasp of your future sales assists you in making well-informed choices and staying ahead of the game. Fortunately, there are several tried-and-tested techniques for forecasting sales effectively. Let us explore three main sales forecasting methods, their methodologies, advantages, and limitations.
Time Series Analysis
Time series analysis is a statistical technique for inspecting and predicting patterns within sequential data points, typically plotted over time. Due to its simplicity and effectiveness, it’s one of the most widely used methods for sales forecasting.
Methodology:
- Data Collection: Gather historical sales data covering a specific time period, typically months or years.
- Data Exploration: Plot the sales data over time to identify trends, seasonality, and irregular patterns.
- Model Selection: Choose a suitable time series model based on the characteristics of the data. Common models include:
- Moving Averages: Calculates the average of a rolling window of sales data to smooth out fluctuations.
- Exponential Smoothing: It gradually reduces the significance of older data points, emphasizing recent observations more through exponential weighting.
- ARIMA (AutoRegressive Integrated Moving Average): Incorporates autoregressive and moving average components to capture complex patterns.
- Model Training: Estimate the chosen model’s parameters with accurate historical data.
- Forecasting: Use the trained model to predict future sales based on extrapolation from past trends.
Advantages:
- Simplicity: Time series analysis doesn’t require complex assumptions or data preprocessing, making it accessible to businesses.
- Flexibility: It can accommodate various types of sales data, including those with seasonal fluctuations or irregular patterns.
- Interpretability: The results are easy to understand and communicate, making it valuable for decision-makers across different departments.
Limitations:
- Assumption Of Stationarity: Time series models assume that the underlying patterns remain constant, which may not always hold in real-world scenarios.
- Sensitivity To Outliers: Extreme data points or anomalies can distort the forecasts, especially in models like moving averages.
- Limited Predictive Power: While practical for short to medium-term forecasts, time series analysis may struggle with long-term predictions or sudden market shifts.
Regression Analysis
Regression analysis is a statistical method that recognizes relationships between a dependent variable (e.g., sales) and one or more independent variables (e.g., advertising expenditure, market demographics). By analyzing historical data, regression models can quantify the impact of different factors on sales and make predictions accordingly.
Methodology:
- Data Collection: Gather historical sales data along with relevant independent variables.
- Data Preprocessing: Clean the data, handle missing values, and ensure compatibility between variables.
- Model Selection: Choose an appropriate regression model based on the nature of the data and the relationship between variables. Common models include:
- Linear Regression: Assumes a linear relationship between the dependent and independent variables.
- Multiple Regression: Extends linear regression to include multiple independent variables.
- Logistic Regression: Used for binary outcomes, such as sales vs. no sales.
- Model Training: Estimate the regression equation coefficients using ordinary least squares (OLS) or maximum likelihood estimation (MLE) techniques.
- Validation: Assess the model’s performance using R-squared, mean squared error (MSE), or cross-validation.
- Forecasting: Utilize the trained regression model to predict future sales based on the values of independent variables.
Advantages:
- Versatility: Regression analysis can accommodate various data types and model complex relationships between variables.
- Quantitative Insights: By examining the regression equation coefficients, businesses can identify which factors have the most significant impact on sales.
- Scalability: Once the model is built, it can generate forecasts quickly and efficiently for different scenarios.
Limitations:
- Assumption of Linearity: Regression models assume a linear relationship between the dependent and independent variables, which may not always be accurate.
- Overfitting: Complex regression models with too many variables risk overfitting the data, leading to poor generalization of new observations.
- Data Requirements: Regression analysis requires significant historical data, especially when dealing with multiple independent variables, which may not always be available for new businesses or niche markets.
Machine Learning (ML) Algorithms
Machine learning algorithms have gained prominence in sales forecasting due to their ability to handle large datasets, nonlinear relationships, and complex patterns. By leveraging advanced techniques such as neural networks, decision trees, and ensemble methods, ML models can often outperform traditional statistical methods in accuracy and flexibility.
Methodology:
- Feature Selection: Identify relevant features (variables) that may influence sales, such as marketing campaigns, economic indicators, or customer demographics.
- Data Preprocessing: Clean the data, handle missing values, and scale numerical features to ensure uniformity.
- Model Selection: Choose an appropriate ML algorithm based on the problem at hand and the characteristics of the data. Common algorithms include:
- Neural Networks: Deep learning models that mimic the structure and function of the human brain, capable of learning complex patterns from data.
- Random Forest: An ensemble learning technique that combines multiple decision trees to improve predictive accuracy and robustness.
- Gradient Boosting: Builds a series of weak learners sequentially, each correcting the errors of its predecessor, leading to predictive solid performance.
- Model Training: Split the data into training and validation sets, train the chosen ML model on the training data, and tune hyperparameters to optimize performance.
- Validation: Evaluate the model’s performance using accuracy, precision, recall, or area under the ROC curve (AUC).
- Forecasting: Deploy the trained ML model to predict new or unseen data, adjusting forecasts as new information becomes available.
Advantages:
- Nonlinearity: ML algorithms can capture nonlinear relationships between variables, offering more accurate forecasts in complex scenarios.
- Feature Importance: ML models can automatically identify the most influential features, providing insights into the drivers of sales.
- Adaptability: ML models can adapt to changing market conditions and evolving trends, making them suitable for dynamic environments.
Limitations:
- Complexity: Building and training ML models requires specialized data science and machine learning expertise, which may not be readily available to all businesses.
- Data Dependency: ML algorithms rely heavily on the quality and quantity of data, requiring large datasets for optimal performance.
- Interpretability: Some ML models, such as deep neural networks, are often regarded as “black boxes” due to their complex internal workings, making it challenging to interpret how predictions are made.
How To Choose The Right Technique For Your Business?
Selecting the appropriate sales forecasting technique for your business requires a thorough understanding of your industry, market dynamics, and available data sources. Begin by assessing the nature of your product or service, the level of historical sales data you possess, and the resources and expertise at your disposal.
Consider the accuracy and reliability required for your forecasts and the timeline for decision-making. Tailor your approach based on the specifics of your business environment and strategic goals. By aligning the chosen forecasting technique with your unique needs and constraints, you can enhance the effectiveness of your sales predictions and make informed decisions to drive growth and profitability.
Implementing The Chosen Sales Forecasting Technique
Implementing the chosen sales forecasting technique is critical to maximizing its effectiveness. Start by organizing and cleaning your historical sales data to ensure accuracy. Utilize advanced analytical tools and software to streamline forecasting and leverage predictive modeling techniques. Collaborate with cross-functional teams to gather insights and validate assumptions for more robust forecasts.
Regularly monitor and adjust your projections based on market trends and performance feedback. Continuous improvement is vital to refining your forecasting capabilities and achieving sustainable business growth.
Monitoring And Adjusting Your Forecasts
Once you have implemented your chosen sales forecasting technique, the next crucial step is constantly monitoring and adjusting your forecasts. By regularly reviewing your data against actual sales performance and market trends, you can identify any discrepancies and refine your forecasting model.
The continuous monitoring process will enable you to stay agile and responsive to changes in the business environment, ensuring that your forecasts remain accurate and reliable. Be proactive in seeking feedback from cross-functional teams and stakeholders to validate assumptions and incorporate new insights into your forecasting process. Stay committed to refining your forecasts to drive sustainable business growth and success.
Frequently Asked Questions
Q1. What is sales forecasting?
Ans. Sales forecasting predicts future sales performance based on historical data, market trends, and other relevant factors. It helps businesses plan operations, allocate resources, and set marketing goals.
Q2. Why is sales forecasting important?
Ans. Sales forecasting is crucial for businesses to make informed decisions regarding production, inventory management, staffing, and budgeting. It provides insights into future revenue streams and helps in setting achievable targets.
Q3. Which sales forecasting technique is the most accurate?
Ans. The accuracy of sales forecasting techniques depends on various factors, including the nature of the business, the availability of data, and the expertise of the analysts. Generally, combining quantitative and qualitative methods produces more accurate forecasts than relying on a single technique.
Q4. What data is needed for sales forecasting?
Ans. Sales forecasting requires historical sales data, market data, economic indicators, customer demographics, and other relevant information. The availability and quality of data significantly impact forecast accuracy.
Conclusion
Sales forecasting is a key aspect of business planning and decision-making. By employing the proper forecasting techniques, organizations can gain valuable insights into future sales trends, mitigate risks, and capitalize on opportunities in the marketplace. Whether using time series analysis, qualitative methods, or quantitative models, businesses must adapt their approach to suit the unique characteristics of their industry, market, and product offerings.
By combining data-driven insights with expert judgment and market intelligence, businesses can enhance the accuracy and reliability of their sales forecasts, enabling them to stay ahead of the competition and achieve sustainable growth in today’s dynamic business environment.
No Comments